Selected Publications
For students interested in our group, please read these SPs before talking to me.
-
Identification of upstream transcription factor binding sites in orthologous genes using mixed Student’s t-test statistics
Locating transcription factor-binding (TF-binding) site in the genome and identification their function is fundamental in understanding various biological processes. Improve the performance of the prediction tools is important because accurate TF-binding site prediction can save cost and time for wet-lab experiments. Also, genome wide TF-binding site prediction can provide new insights for transcriptome regulation in system biology perspective. This study developed a new TF-binding site prediction tool based on mixed Student’s t-test statistical method. The tool is amongst the top-ranked TF-binding site predictors, as such, it can help the researchers in TF-binding site identification and transcriptional regulation mechanism interpretation of genes.
-
GEREA: prediction of gene expression regulators from transcriptome profiling data to transition networks
Background: Mammalian genes are regulated at the transcriptional and post-transcriptional levels. These mechanisms may involve the direct promotion or inhibition of transcription via a regulator or post-transcriptional regulation through factors such as microRNAs. Objective: Build genes regulation relationships modulated by causality inference-based microRNA-(transition factor)-(target gene) networks and analysis gene expression data to find gene expression regulators. Methods: Manually curate mouse gene expression regulation relationships from the literature using text mining method, and built microRNA-(transition factor)-(target gene) networks. Identifying gene expression regulators from transcriptome profiling data by applying enrichment analysis to these networks. Results: A total of 22,271 mouse gene expression regulation relationships have been curated for 4,018 genes and 242 microRNAs. A software called GEREA were developed to perform the integrated analyses. We applied the algorithm to transcriptome data for synthetic miR-155 oligo-treated mouse CD4+ T-cells and confirmed that miR-155 was an important network regulator. Wet-lab experiments verified that miR-155 regulates the transition factors Sp1, Fgf2, and Ctla4. An in vitro analysis of target transcription levels in transition factor inhibitor-treated mouse CD4+ T-cells determined the regulatory effects of the two transition factors. Conclusion: The causality inference-based microRNA-(transition factor)-(target gene) networks is a novel resource for gene expression regulation research, and GEREA is an effective and useful adjunct to the currently available methods. The regulatory networks and the algorithm implemented in the GEREA software package are available under a free academic license at http://www.thua45.cn/gerea.
-
GEREDB: Gene expression regulation database curated by mining abstracts from literature
Understanding how genes are expressed and regulated in di®erent biological processes are fundamental and challenging issues. Considerable progress has been made in studying the relationship between the expression and regulation of human genes. However, it is di±cult to use these resources productively to analyze gene expression data. GEREDB (www.thua45.cn/geredb) has been developed to facilitate analyses that will provide insights into the regulation of genes that govern speci¯c biological responses. GEREDB is a publicly available, manually curated biological database that stores the data regarding relationships between expression and regulation of human genes. To date, more than 39,000 Links have been contextually annotated by reviewing more than 53,000 abstracts. GEREDB can be searched using the o±cial NCBI gene symbol as a query, and it can be downloaded along with the GEREA software package. GEREDB has the ability to analyze user-supplied gene expression data in a causal analysis oriented manner using the GEREA bioinformatics tool.
Read Full Text Journal of Bioinformatics and Computational Biology Paper
-
Min3: Predict microRNA target gene using an improved binding-site representation method and support vector machine
MicroRNAs are single-stranded noncoding RNAs known to down-regulate target genes at the protein or mRNA level. Computational prediction of targets is essential for elucidating the detailed functions of microRNA. However, prediction speci¯city and sensitivity of the existing algorithms still need to be improved to generate useful hypotheses for subsequent experimental testing. A new microRNA binding-site representation method was developed, which uses four symbols \j", \:", \", and \ ^" (indicating paired, unpaired, insertion, and bulge, respectively) to represent the status of each nucleotide base pair in the microRNA binding site. New features were established with the information of every two adjacent symbols. There are 12 possible combinations and the frequency of each de¯nes a set of novel and useful features. A comprehensive training dataset is constructed for mammalian microRNAs with positive targets obtained from the microRNA target depository in the miRTarbase, while negative targets were derived from pseudo-microRNA bindings. An SVM model was established using the training dataset and a new software called Min3 was developed. Performance of Min3 was assessed with intensively studied examples of miR-155 and miR-92a. Prediction results showed that Min3 can discover 47% of experimental conformed targets on average. The overlapping is above 20% on average when compared with TargetScan and miRanda. Annotations of the public microRNA datasets showed that there is a negative e®ect (up-regulation) of the Min3 targets for the knock out/down of miR-155 and miR-92a. Six top ranked targets were selected for validation by wetlab experiments, and ¯ve of them showed a regulation e®ect. The Min3 can be a good alternative to current microRNA target discovery software. This tool is available at https://sourceforge. net/projects/mirt3.
Read Full Text Journal of Bioinformatics and Computational Biology Paper
-
K-walks: clustering gene-expression data using a K-means clustering algorithm optimised by random walks
Gene-expression data obtained from the biological experiments always have thousands of dimensions, which can be very confusing and perplexing to biologists when viewed as a whole. Clustering analysis is an explorative data-mining technique for statistical data analysis that is widely used in gene-expression data analysis. Practical approaches employed for solving the clustering problem use iterative procedures such as K-means, which typically converge to one of many local minima. Here, we propose a simulated annealing approximation algorithm that is optimised using random walks to solve the K-means clustering problem. The algorithm is verified with synthetic and real-world data sets and compared with other well-known K-means variants. The new algorithm is less sensitive to initial cluster centres, and the primary strength of our algorithm is its ability to produce high-quality clustering results for thousands of high-dimensional data. However, the algorithm is computationally intensive.
-
MiRFinder: an improved approach and software implementation for genome-wide fast microRNA precursor scans
Background: MicroRNAs (miRNAs) are recognized as one of the most important families of noncoding RNAs that serve as important sequence-specific post-transcriptional regulators of gene expression. Identification of miRNAs is an important requirement for understanding the mechanisms of post-transcriptional regulation. Hundreds of miRNAs have been identified by direct cloning and computational approaches in several species. However, there are still many miRNAs that remain to be identified due to lack of either sequence features or robust algorithms to efficiently identify them. Results: We have evaluated features valuable for pre-miRNA prediction, such as the local secondary structure differences of the stem region of miRNA and non-miRNA hairpins. We have also established correlations between different types of mutations and the secondary structures of pre-miRNAs. Utilizing these features and combining some improvements of the current premiRNA prediction methods, we implemented a computational learning method SVM (support vector machine) to build a high throughput and good performance computational pre-miRNA prediction tool called MiRFinder. The tool was designed for genome-wise, pair-wise sequences from two related species. The method built into the tool consisted of two major steps: 1) genome wide search for hairpin candidates and 2) exclusion of the non-robust structures based on analysis of 18 parameters by the SVM method. Results from applying the tool for chicken/human and D. melanogaster/D. pseudoobscura pair-wise genome alignments showed that the tool can be used for genome wide pre-miRNA predictions. Conclusion: The MiRFinder can be a good alternative to current miRNA discovery software. This tool is available at http://www.bioinformatics.org/mirfinder/.
Happy Clients
Projects
Years of experience
Awards
The mission of Tinghua Huang's bioinformatics laboratory
Further advance the interdisciplinary field of bioinformatics by conducting internationally recognized bioinformatics research in the broad areas of DNA and protein sequence analysis; Provide graduate-level bioinformatics educational training to produce graduates competitive at the regional, national, and international levels.
- Computational analysis of gene regulatory regions.
- Systems biology approaches for gene expression meta-analysis
- Algorithmic development for analysis of Next-Generation sequencing data
The development of high throughput technologies has given rise to a wealth of information at system level including genome, epigenome, transcriptome, proteome and metabolome. However, it remains a major challenge to analysis the massive amounts of information and use it in an intelligent and comprehensive manner. To address this question, Dr. Tinghua Huang's group has focused on developing computational tools and resources to analyze and integrate large scale 'omics' datasets, which help researchers to understand how genes work together to comprise a specific biological process.
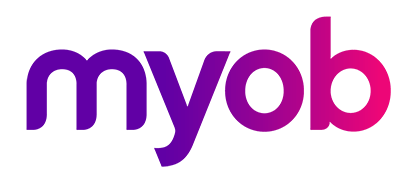

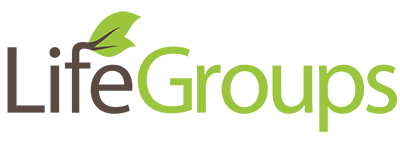
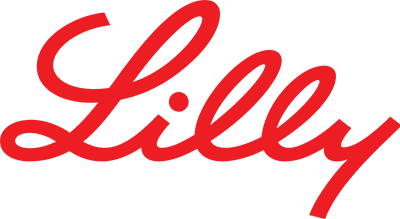
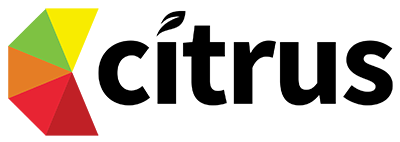
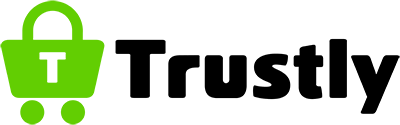
Sevices
Our full Services and Information Catalog is your guide to Tinghua Huang's bioinformatics group.
Call To Action
Read our Papers with these big influencers of our tools on experment design, and get 100% free plan. Learn more about this here.
Install NowMembers
Students who are currently enrolled at Yangtze University Tinghua Huang's group can take advantage of our academic advising and use these pages to find information and resources. We encourage prospective and newly admitted students to browse this information as part of the application process and as you prepare to register for classes.
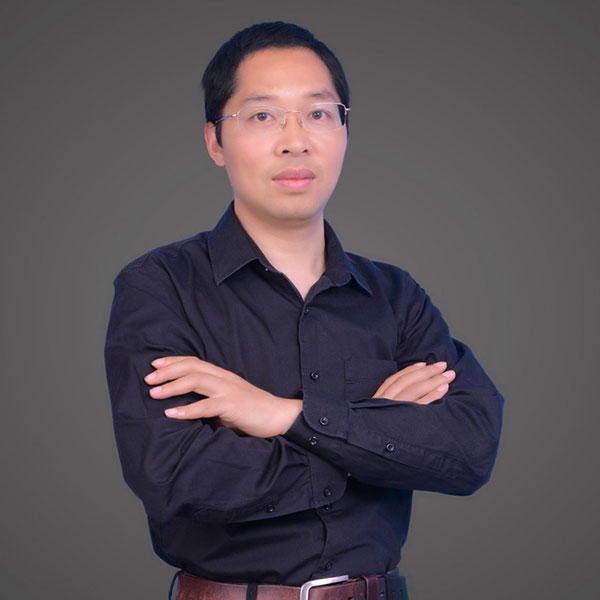
Tinghua Huang
Suppervisor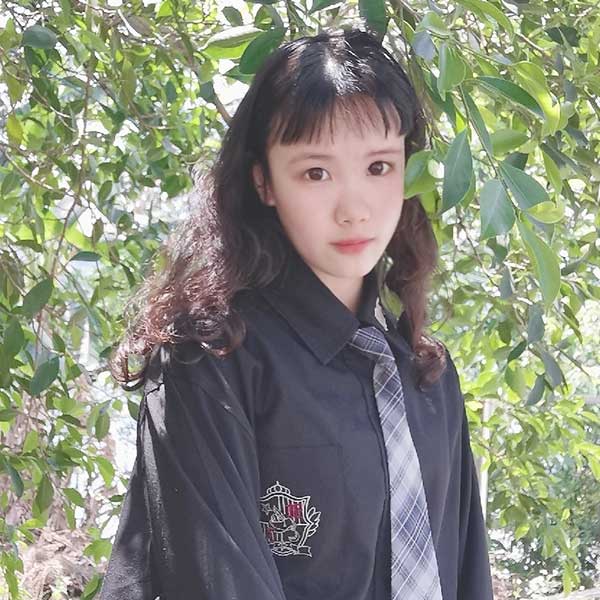
Hong Xiao
Undergraduate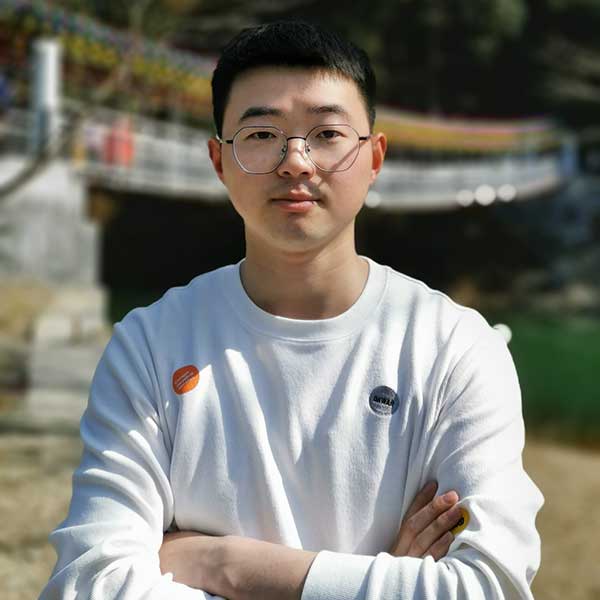
Qi Tian
Undergraduate
Zhen He
UndergraduatePlan
Our tool is available in open source and runs on the desktop (Windows, Mac, and Linux) or in a browser connected to our Server (Debian/Ubuntu, Red Hat/CentOS, and SUSE Linux).
FAQ for Students
For students interested in our group, please read this FAQ before talking to me.
-
Will I get financial support if joining the group?
In general, yes (including undergraduate students).
-
It seems the group uses a lot of mathematics. Do I have enough backgrounds?
Yes. We don't expect you to have such knowledge. However, for any new materials, you must be able to learn them by yourselves. BTW, we run many experiments, so programming ability is also very important.
-
Does the group mainly use unix-type computers for programming?
Yes. Our members might not be familiar with such systems in the beginning, but they quickly catch up (by themselves).
-
What is really needed for being a member of this group?
Creativity to think about different directions and courage to try things.
-
Is it necessary to work very hard?
Definitely not. We judge you by your research results but not by how hard you have worked.
-
So roughly speaking what type of students are very suitable for this group?
Those who are enthusiastic about doing research. In general, if you would like to be a great researcher in the future, then this group is more suitable for you.
-
Is there any difference for PhD, master, and undergraduate students in the group?
No. They are all treated as PhD students.
-
Will you give me a specific problem to work on?
Yes and no. Good research problems come from active discussion. As I am more experienced than you, through discussion I may be able to identify some good topics for you. So if no discussion, no good topics.
-
I am an undergraduate student. Is it possible to finish some great work in my senior year?
Possible but a bit difficult. So usually we encourage undergraduate students to join the group as early as possible.
-
I am an undergraduate student in the group. May I assume that I can stay in the group for my graduate study?
No. You need to talk to me first. Otherwise, we assume that you are leaving the group after your undergraduate study.
-
For any paper I write, must my advisor be a co-author?
No. The authorship of a paper is related to the contribution rather than the group you are associated with. Here are a few situations that you may write a paper without my name on it. 1) Without my guidence, you finish some excellent work and write a paper. Then of course I shouldn't be a co-author. I always hope that this happens sometime, but so far our students need some my help on both the research work as well as writing. 2) You work with another group and write a paper with them. If I am not involved in this work, then of course I shouldn't be a co-author.
-
I would like to audit in your group meeting. Is this ok?
You are very welcome.
-
Will I be allowed to play games in the lab?
Yes at any time. We hope you feel like at your home.
-
There is a big snoopy in the lab. Could I hold her and sleep on the sofa?
The same as the previous question. But you may have found her sitting on my lap.